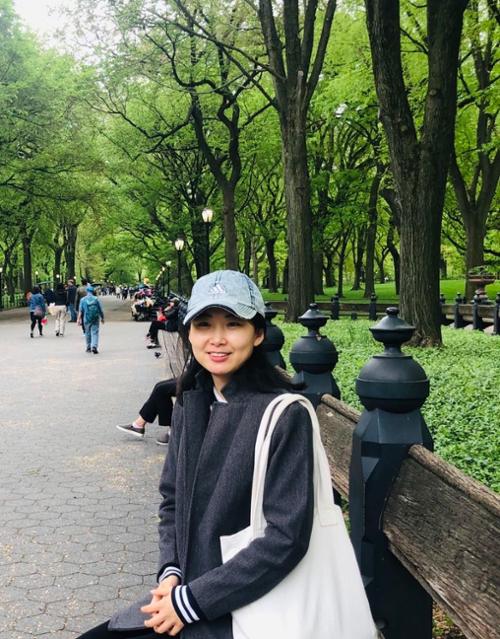
Yan Cong
Assistant Professor
// SLC
Faculty
Assistant Professor
// Chinese // SLC
Faculty
Assistant Professor
// Cornerstone
Faculty
Research focus:
Chinese and Computational Linguistics
Office and Contact
Room: SC G015
Email: cong4@purdue.edu
Yan Cong's work focuses on language in humans and machines. Yan works in the areas of natural language processing (NLP), semantics and pragmatics, and speech and language technology. Yan is currently developing text analysis models to quantify, understand, and improve language learning.
Previously Yan was an NLP researcher at the Feinstein Institutes. Before that, Yan completed her PhD in linguistics from Michigan State University.
Specializations
Assessment of semantic/pragmatic competence in (Large) Language Models, Chinese linguistics, Computational approach to speech and language fluency
Affiliation
I originally came from a background in linguistics, interested in language in humans, specifically how humans understand language in context. I use formal derivations to model meaning, its interaction with structure, and how speakers and listeners reason about meaning. Fairly early on I developed a strong interest in both using computational methods to explore linguistic questions and applying puzzles in language to the design of AI systems.
This is what sent me in this direction of being what I am today, which is simultaneously someone who works on natural language processing (NLP) and AI, and someone who continues to work on computational modeling pertaining to language, using computational methods to justify and generalize linguistic theories, and making better predictions about the possibilities and the impossibilities in language.
In general, my core interests are the science of language. But I am also motivated by potential applications. I hope to put what we know to good use and provide options for students. I am interested in translating NLP and AI methods to other fields such as language education and healthcare, directly helping learners, teachers, and practitioners.
In particular, I use linguistic frameworks to assess the linguistic capacities of AI systems, teasing "understanding" apart from shallower heuristic strategies, and improving AI systems' flexibility and transparency. An interpretable AI system also serves as a reliable and efficient tool to understand and improve language learning. To that end, I am committed to developing robust AI systems in language research and applied linguistics contexts.